Predictive and prescriptive analytics are two forward-looking tools used by business leaders which overcome these limitations. Using a combination of historical data (descriptive analytics), rules and a knowledge of the business, they more accurately predict the future, and, in the case of prescriptive analytics, guide leaders to the best overall decisions.
The growing importance of these analytical techniques is reflected by a prediction that the global market for predictive and prescriptive analytics will grow at a CAGR of 19.6 percent between 2017 and 2026 to a total of $28.7 billion.
Is There a Difference Between Predictive and Prescriptive Analytics, or Are They Essentially the Same?
Although both techniques are forward-looking, there’s a distinct difference between the two. This can be seen from Gartner’s Analytic Ascendency Model, which defines the hierarchy of analytical techniques as follows:
- Descriptive analytics: What happened?
- Diagnostic analytics: Why did it happen?
- Predictive Analytics: What will happen?
- Prescriptive Analytics: How can we make it happen?
While predictive analytics gives an indication of what may happen, prescriptive analytics shows the way to make it happen. In this context, predictive analytics is subordinate to prescriptive analytics. This is not to say that predictive analytics are inferior, simply that the type of information provided is different.
Predictive analytics can be defined as the use of statistical and modeling techniques to determine what may happen in the future. It uses historical data and modeling techniques to arrive at a likelihood of a specific event or events. A good example of predictive analysis is predictive maintenance, which attempts to determine when a machine needs servicing using various algorithms and measured machine data to estimate the life of critical components. Although this information is useful and actionable, in and of itself it doesn’t determine what action should be taken, it simply informs the user that maintenance is needed.
Prescriptive analytics differs in that it not only identifies what’s likely to happen, but also presents the user with specific options and determines which business options are best in terms of certain criteria. With prescriptive analytics, a model of the business or organization is created. This model is validated against current and historical data to ensure it accurately represents all facets of the business. Instead of simply predicting what will happen, users can interrogate the model to establish the best decision according to predefined criteria such as profitability, SLAs and throughput. In the context of the predictive maintenance example above, prescriptive analytics not only identifies that maintenance is needed, but it determines the optimal decisions regarding maintenance, replacement or outsourcing to maximize overall profitability and turnover.
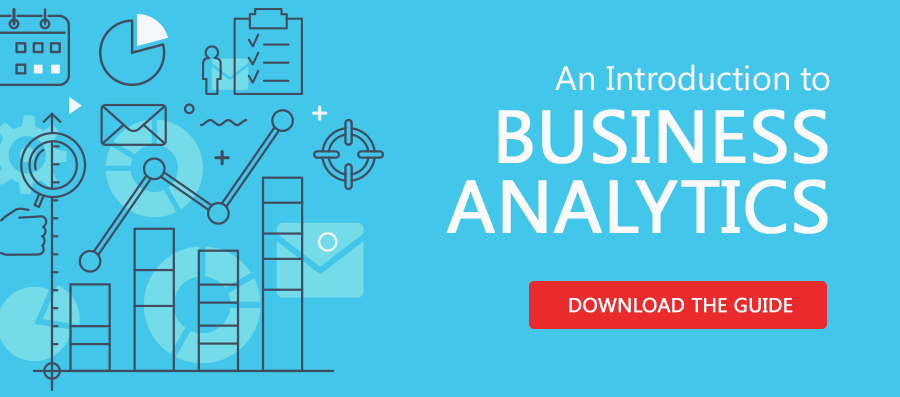
Key Differences Between Predictive and Prescriptive Analytics
Predictive and prescriptive analytics are both important business tools, and each has a role to play. But in terms of Gartner’s analytics hierarchy referred to earlier, predictive analytics is inferior to prescriptive analytics. This is because predictive analytics indicates what will happen but gives no guidance as to decisions that need to be made. On the other hand, prescriptive analytics not only determines what will happen, it also establishes the best decision for the business.
Predictive analytics
- Models certain aspects of a business
- Forecasts what’s likely to happen
- Predicts when it will happen
- Outputs are non-actionable; they only identify the need to take a decision
- Tends to optimize one function at the expense of others
- Usually based on hypotheses using predetermined scenarios with finite options
Prescriptive Analytics
- Models the entire business
- Is 100 percent data driven
- Recommends specific business decisions
- Considers interdependencies
- Is not bound by static rules
- Provides tangible, measurable benefits
- Supports what-if scenarios
- Is free of “gut feel” and personal bias
- Accounts for all inputs, variables and outputs
- Uses calibrated and validated models that truly reflect how the business operates
YES: The Difference Between Predictive and Prescriptive Analytics Matters
Some may ask whether the difference between these two analytics matters in practical terms. They may also point to the fact that there’s clearly a lot more work to optimize a prescriptive analytics solution than for a smaller scale predictive analytics experiment. In answering that question, it’s important to consider the business maturity of prospective customers.
While most businesses use business intelligence, not all have progressed to predictive analytics. In fact, according to Gartner, only 11 percent of medium to large businesses are currently using prescriptive analytics. However, Gartner anticipates the prescriptive analytics software market will experience a 20.6 percent CAGR between 2017 and 2022. This suggests that nearly 37 percent of business will start using prescriptive analytics.
For a growing number of companies, these figures indicate the difference between predictive and prescriptive analytics does matter.
Although predictive analytics excels at identifying potential problems and challenges, only prescriptive analytics ascertains the best way forward to maximize business opportunities. The two analytical techniques fulfill totally separate needs. Predictive analytics are reactive in that they highlight the need for management to react. Conversely, prescriptive analytics are proactive in that they show management the way forward.
Predictive and prescriptive analytics work with real data a business captures, along with other available information. The key difference is that predictive analytics simply interprets trends, whereas prescriptive analytics uses heuristics (rules)-based automation and optimization modeling to determine the best way forward.
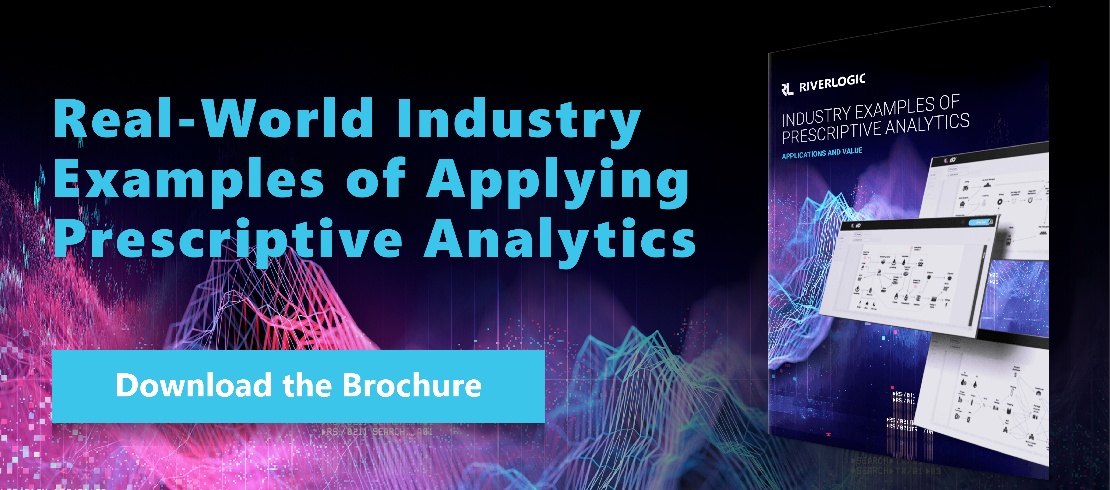
Problems You’d Tackle with Predictive Versus Prescriptive Analytics
Predictive and prescriptive analytics are not stand-alone tools used in isolation. There’s a place within organizations for all forms of business analytics to solve different problems.
Predictive analytics are generally used to identify short- to medium-term trends, often in isolation of other broader trends. Typical examples include:
- Sales trends including those of specific lines and products
- Short-term insurance risk analysis
- Demand planning
- Inventory control
- Profitability
- Maintenance requirements
- Customer churn
Prescriptive analytics looks at the bigger picture. While predictive analytics may measure individual trends, prescriptive models usually evaluate entire businesses, or at the very least, discrete functions, divisions or plants. Examples of problems solved using prescriptive analytics include:
- Optimizing coal extraction over a number of mines to simultaneously meet customer requirements and increase overall profitability.
- Establishing an optimal manufacturing and inventory strategy for consumer goods companies.
- Determining the best operation strategy for a waste water treatment utility covering a large metropolitan area while maintaining regulatory compliance.
The Difference in Value That Both Techniques Bring to the Organization
Although both methods offer tangible benefits, the results from prescriptive analytics usually far outweigh those from predictive analytics. While this is due in part to the scale of operations, it’s also affected by the types of decisions made as well as the ability of prescriptive analytics to optimize decisions.
Predictive analytics tend to focus on a relatively narrow set of parameters, such as the example above, for short-term risk analysis. While this type of analysis can result in huge rewards by limiting risk, it’s unlikely to be in the same order of magnitude as a prescriptive analytics solution that models the insurance company’s business. Such a model can identify the most profitable insurance products, pinpoint the best markets and identify optimal strategies for sustained business growth. Additionally, business executives can use prescriptive analytics to explore multiple what-ifs, options and trade-offs without being limited to predetermined scenarios.
While prescriptive solutions are likely to be more expensive than predictive ones, the ROI of prescriptive analytics has the potential to be far greater than with predictive analytics. This is illustrated in the coal mining example above where the company increased its annual revenue by 4 percent ($250 million) while achieving 100 percent customer satisfaction.
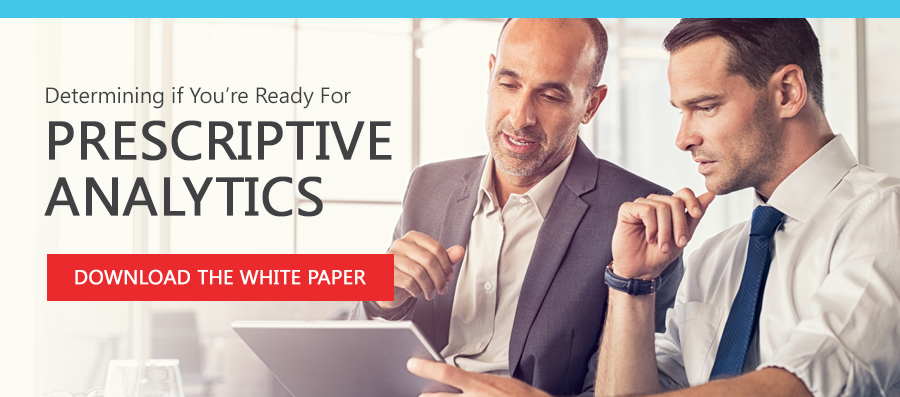
The Difference in Technology Requirements
Traditionally, data analysis was the preserve of data scientists; however, the fast pace of business today makes it imperative that line managers and executives have direct access to these analytical tools. While this doesn’t mean they should be involved in programming and data cleansing, it does imply the provision of end-user tools and dashboards that allow them to interrogate results themselves. This hands-on approach builds confidence in the tools as well as presenting on-the-spot information to support decision-making.
The various tools available for prescriptive analytics vary from high-level programming languages to built-in ERP tools and solution-specific software packages. The first step is to clean up and combine the data so it’s usable. Next, various analytical techniques are applied, such as:
- Regression techniques, such as linear, time-based and logistic regression
- Machine learning techniques
- Neural networks
- Naïve Bayes conditional probability
Prescriptive analytics takes this a step further using one of two types of analysis: heuristics and optimization.
Heuristics are used for operational situations that can be narrowly defined. This technique is a rules-based mathematical approach. It’s good in situations where similar decisions are made frequently, such as with raw material purchasing. Heuristics are good for automated decisions but aren’t as suitable for optimizing decisions. Weaknesses include the need for the constant revision of rules to avoid them becoming out of date and that heuristics can’t analyze every possible scenario.
Optimization uses a combination of mathematical models and exact algorithms that determine the optimal solution. A mathematical model representing the business or function is developed, and an exact algorithm is used to answer specific questions. The objective of an optimization model is to maximize or minimize a parameter, such as profit or costs.
Prescriptive analytics software is available as packaged solutions or as optimization platforms. Packages have the advantage of being easier to configure and are often written to solve a common problem or for a specific industry. They’re usually available through the cloud as SaaS or PaaS solutions.
Optimization platforms have two components: a modeling platform, where the problem is defined, and an optimization solver. Models are written mathematically or by means of a drag-and-drop visual interface. Optimization platforms usually run in-house and costs vary enormously. They offer highly customized solutions that accurately represent the problem. They may require expert programmers to construct the model and often don’t have end-user interfaces. Some require advanced programing skills, while others, such as River Logics Microsoft Azure platform, don’t.
Prescriptive Analytics Guide to Long-term Decision-making
The difference between predictive and prescriptive analytics is that the former provides short term metrics that help understand what’s happening in the organization, whereas the latter provides answers to what should be done.
Predictive analytics measure metrics in isolation, but don’t evaluate their overall impact. For example, they can measure and predict an organization’s sales performance but won’t necessarily measure the impact of increased raw material costs on cost of sales and profitability.
Prescriptive analytics model businesses while taking into account all inputs, processes and outputs. Models are calibrated and validated to ensure they accurately reflect business processes. Prescriptive analytics recommend the best way forward with actionable information to maximize overall returns and profitability. Prescriptive analytics models support informed decision-making.
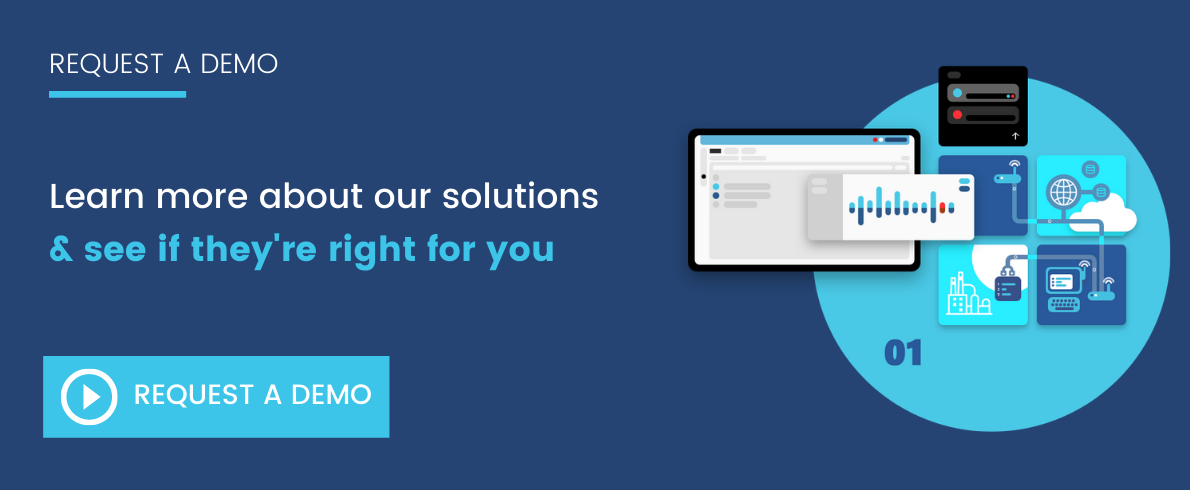